TechDispatch #1/2021 - Facial Emotion Recognition
Facial Emotion Recognition (FER) is the technology that analyses facial expressions from both static images and videos in order to reveal information on one’s emotional state. The complexity of facial expressions, the potential use of the technology in any context, and the involvement of new technologies such as artificial intelligence raise significant privacy risks.
1. What is Facial Emotion Recognition?
Facial Emotion Recognition is a technology used for analysing sentiments by different sources, such as pictures and videos. It belongs to the family of technologies often referred to as “affective computing”, a multidisciplinary field of research on computer’s capabilities to recognise and interpret human emotions and affective states and it often builds on Artificial Intelligence technologies.
Facial expressions are forms of non-verbal communication, providing hints for human emotions. For decades, decoding such emotion expressions has been a research interest in the field of psychology (Ekman and Friesen 2003; Lang et al. 1993) but also to the Human Computer Interaction field (Cowie et al. 2001; Abdat, Maaoui, and Pruski 2011). Recently, the high diffusion of cameras and the technological advances in biometrics analysis, machine learning and pattern recognition have played a prominent role in the development of the FER technology.
Many companies, ranging from tech giants such as NEC or Google to smaller ones, such as Affectiva or Eyeris invest in the technology, which shows its growing importance. There are also several EU research and innovation program Horizon2020 initiatives1 exploring the use of the technology.
FER analysis comprises three steps: a) face detection, b) facial expression detection, c) expression classification to an emotional state (Figure 1). Emotion detection is based on the analysis of facial landmark positions (e.g. end of nose, eyebrows). Furthermore, in videos, changes in those positions are also analysed, in order to identify contractions in a group of facial muscles (Ko 2018). Depending on the algorithm, facial expressions can be classified to basic emotions (e.g. anger, disgust, fear, joy, sadness, and surprise) or compound emotions (e.g. happily sad, happily surprised, happily disgusted, sadly fearful, sadly angry, sadly surprised) (Du, Tao, and Martinez 2014). In other cases, facial expressions could be linked to physiological or mental state of mind (e.g. tiredness or boredom).
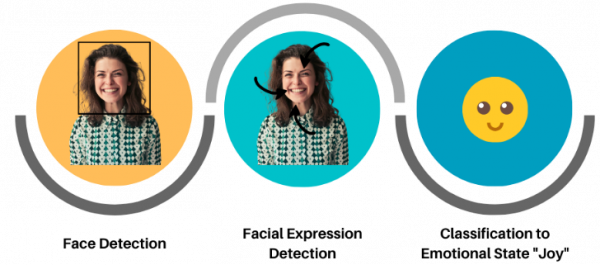
Figure 1: Steps of Facial Emotion Recognition
The source of the images or videos serving as input to FER algorithms vary from surveillance cameras to cameras placed close to advertising screens in stores as well as on social media and streaming services or own personal devices.
FER can also be combined with biometric identification. Its accuracy can be improved with technology analysing different types of sources such as voice, text, health data from sensors or blood flow patterns inferred from the image.
Potential uses of FER cover a wide range of applications, examples of which are listed here below in groups by their application field.
Provision of personalised services
- analyse emotions to display personalised messages in smart environments
- provide personalised recommendations e.g. on music selection or cultural material
- analyse facial expressions to predict individual reaction to movies
Customer behaviour analysis and advertising
- analyse customers’ emotions while shopping focused on either goods or their arrangement within the shop
- advertising signage at a railway station using a system of recognition and facial tracking for marketing purposes
Healthcare
- detect autism or neurodegenerative diseases
- predict psychotic disorders or depression to identify users in need of assistance
- suicide prevention
- detect depression in elderly people
- observe patients conditions during treatment
Employment
- help decision-making of recruiters
- identify uninterested candidates in a job interview
- monitor moods and attention of employees
Education
- monitor students’ attention
- detect emotional reaction of users to an educative program and adapt the learning path
- design affective tutoring system
- detect engagement in online learning
Public safety
- lie detectors and smart border control
- predictive screening of public spaces to identify emotions triggering potential terrorism threat
- analysing footage from crime scenes to indicate potential motives in a crime
Crime detection
Other
2. What are the data protection issues?
Due to its use of biometric data and Artificial Intelligence technologies, FER shares some of the risks of using facial recognition and artificial intelligence. Nevertheless, this technology carries also its own specific risks. Being a biometrics technology, where aiming at identification does not appear as a primary goal, risks related to emotion interpretation accuracy and its application are eminent.
2.1 Necessity and proportionality
Turning human expressions into a data source to infer emotions touches clearly a part of peoples’ most private data. Being a disruptive technology, FER raises important issues regarding necessity and proportionality.
It has to be carefully assessed, whether deploying FER is indeed necessary for achieving the pursued objectives or whether there is a less intrusive alternative. There is risk of applying FER without performing necessity and proportionality evaluation for each single each case, misled by the decision to use the technology in a different context. However proportionality depends on many factors, such as the type of collected data, the type of inferences, data retention period, or potential further processing.
2.2 Data accuracy
Analysis of emotions based on facial expressions may not be accurate, as facial expressions can slightly vary among individuals, may mix different emotional states experienced at the same time (e.g. fear and anger, happy and sad) or may not express an emotion at all. On the other hand, there are emotions that may not be expressed on someone’s face, thus inference based solely on facial expression may lead to wrong impressions. Additional factors can add to the ambiguity of the facial expressions, such as contextual clauses (sarcasm), and socio-cultural context. In addition, technical aspects (different angles of the camera, lighting conditions and masking several parts of the face) can affect the quality of a captured facial expression.
Furthermore, even in the case of accurate recognition of emotions, the use of the results may lead to wrong inferences about a person, as FER does not explain the trigger of emotions, which may be a thought of a recent or past event. However, the results of FER, regardless of accuracy limitations, are usually treated as facts and are input to processes affecting a data subject’s life, instead of triggering an evaluation to discover more about their situation in the specific context.
2.3 Fairness
The accuracy of the facial emotion algorithm results can play an important role in discriminating on grounds of skin colour or ethnic origin. Societal norms and cultural differences have been found to influence the level of expression of some emotions while some algorithms have been found to be biased against several groups, based on skin colour. For instance, a study testing algorithms of facial emotion recognition revealed they assigned more negative emotions (anger) to faces of persons of African descent than to other faces. Furthermore, whenever there was ambiguity, the former were scored as angrier (Rhue, 2018).
Choosing the right dataset that is representative is crucial for avoiding discrimination. If the training data is not diverse enough, the technology might be biased against underrepresented population. Discrimination triggered by faulty database or by errors in detecting the correct emotional state may have serious effects, e.g. inability to use certain services.
In another aspect of the same problem, in case of medical conditions or physical impairments in which temporary or permanent paralysis of facial muscles occurs, data subjects’ emotions may be misunderstood by algorithms. This may result in a wide range of situations of misclassification, with impact ranging from receiving unwished services up to misdiagnosis of having a psychological disorder.
2.4 Transparency and control
Facial images and video can be captured anywhere, thanks to the ubiquity and small size of cameras. Surveillance cameras in public spaces or stores are not the only cameras remotely capturing facial images as one’s own mobile devices can capture expressions during their use. In these situations, transparency issues arise concerning both the collection and the further processing of personal data.
Where the data subjects’ facial expressions are captured in a remote manner, it may not be clear to them which system or application will process their data, for which purposes, and who the controllers are. As a result, they would not be in the position to freely give consent or exercise control over the processing of their personal data, including sharing with third-parties. Where data subjects are not provided with accurate information, access and control over the use of FER, they are deprived of their freedom to select which aspects of their life can be used to affect other contexts (e.g. emotions in social interactions could be used in the context of recruitment). Moreover, data subjects need to control which periods of time their captured data will be processed and aggregated to history records of their emotional situation, as emotion inferences may not be valid for them after a period of time.
Another consequence of the remote capture of facial expressions and the obscurity of their processing is that data subjects might not be provided with information on which other sources of data these will be aggregated to. Also, advanced AI algorithms add to the complexity of transparency needs, as they may detect slight movements of facial muscle that are unconscious even for the individuals. This would contribute to the unpleasant feeling of vulnerability due to unwanted exposure.
2.5 Processing of special categories of personal data
FER technology can detect the existence, changes or total lack of facial expressions, and link this to an emotional state. As a result, in some contexts, algorithms may infer special categories of personal data, such as political opinions or health data. For instance, applying FER technology at political events, political attitudes can be inferred by looking at facial expressions and reactions of the audience. Also, by the lack of facial expressions, algorithms are able to detect signs of alexithymia, a state in which one cannot understand the feelings they experience or lack the words to describe these feelings. This finding can be linked to severe psychiatric and neurological disorders, such as psychosis. Furthermore, analysis of historical data on one’s emotional state may reveal other health conditions such as depression. Such data, if used in the context of healthcare, could assist in prediction and timely treatment of a patient. However, where data subjects are not able to control the flow of derived information and its use in other contexts, they may face a situation of inference and use of such sensitive personal data by non-authorised entities, such as employers or insurance companies.
2.6 Profiling and automated decision-making
FER technology can be further used to create profiles of people in a number of situations. It could be used to derive one’s acceptance of a product, an advertisement or a proposed idea. It can also be used for classifying productivity and fatigue-resistance in workplaces. The risk lies in the fact that the data subject may not be aware of this type of targeting and might feel uncomfortable if they found out about it. Further implications can occur by erroneous profiling or inferences solely based on the association with a certain group of people experiencing the same emotions.
In addition, the knowledge of the individuals’ emotions can make it easier to manipulate them. For instance, the knowledge of emotions revealing a vulnerable emotional state, can be used to mentally force people to perform actions they would not do otherwise – e.g. to buy goods they do not need.
FER technology could be used for purposes of safeguarding public security, for instance at concerts, sport events or airports, to quickly identify signs of aggression and stress and identify potential terrorists. However, if such an identification was based solely on FER and was not combined with other actions or triggers that this person is dangerous, this could introduce further risks for the data subjects. For instance, a person could be subject to unjustified delays to perform further security checks or investigations, causing them to miss participation in an event, boarding on a flight or even lead to unjustified arrest.
Last but not least, FER can influence behavioural changes in case a person is aware of the exposure to this technology (known as Reactivity in psychology). Individuals may alter their habits or avoid specific areas where the technology is applied in an attempt to self-sensor and protect themselves. One can imagine the chilling effect this could have to a society and the feeling of insecurity among citizens, if such a technology were to be used by non-democratic governments, to infer political attitude of citizens.
Recommended Reading
Abdat, F., C. Maaoui, and A. Pruski. 2011. “Human-Computer Interaction Using Emotion Recognition from Facial Expression.” In 2011 UKSim 5th European Symposium on Computer Modeling and Simulation. IEEE. https://doi.org/10.1109/ems.2011.20.
Andalibi, Nazanin, and Justin Buss. 2020. “The Human in Emotion Recognition on Social Media: Attitudes, Outcomes, Risks.” In Proceedings of the 2020 Chi Conference on Human Factors in Computing Systems, 1–16. CHI ’20. New York, NY, USA: Association for Computing Machinery. https://doi.org/10.1145/3313831.3376680.
Barrett, Lisa Feldman, Ralph Adolphs, Stacy Marsella, Aleix M. Martinez, and Seth D. Pollak. 2019. “Emotional Expressions Reconsidered: Challenges to Inferring Emotion from Human Facial Movements.” Psychological Science in the Public Interest 20 (1): 1–68. https://doi.org/10.1177/1529100619832930.
Cowie, R., E. Douglas-Cowie, N. Tsapatsoulis, G. Votsis, S. Kollias, W. Fellenz, and J. G. Taylor. 2001. “Emotion Recognition in Human-Computer Interaction.” IEEE Signal Processing Magazine 18 (1): 32–80. https://doi.org/10.1109/79.911197.
Crawford, K., R. Dobbe, T. Dryer, G. Fried, B. Green, E. Kaziunas, A. Kak, et al. 2919. “AI Now 2019 Report.” New York: AI Now Institute. https://ainowinstitute.org/AI_Now_2019_Report.html.
Daily, Shaundra B., Melva T. James, David Cherry, John J. Porter, Shelby S. Darnell, Joseph Isaac, and Tania Roy. 2017. “Affective Computing: Historical Foundations, Current Applications, and Future Trends.” In Emotions and Affect in Human Factors and Human-Computer Interaction, 213–31. Elsevier. https://doi.org/10.1016/b978-0-12-801851-4.00009-4.
Du, Shichuan, Yong Tao, and Aleix M. Martinez. 2014. “Compound Facial Expressions of Emotion.” Proceedings of the National Academy of Sciences 111 (15): E1454–E1462. https://doi.org/10.1073/pnas.1322355111.
Ekman, Paul, and Wallace V Friesen. 2003. Unmasking the Face: A Guide to Recognizing Emotions from Facial Clues. Ishk.
Jacintha, V, Judy Simon, S Tamilarasu, R Thamizhmani, K Thanga yogesh, and J. Nagarajan. 2019. “A Review on Facial Emotion Recognition Techniques.” In 2019 International Conference on Communication and Signal Processing (Iccsp), 0517–21. IEEE. https://doi.org/10.1109/ICCSP.2019.8698067.
Ko, Byoung Chul. 2018. “A Brief Review of Facial Emotion Recognition Based on Visual Information.” Sensors 18 (2): 401. https://doi.org/10.3390/s18020401.
Lang, Peter J., Mark K. Greenwald, Margaret M. Bradley, and Alfons O. Hamm. 1993. “Looking at Pictures: Affective, Facial, Visceral, and Behavioral Reactions.” Psychophysiology 30 (3): 261–73. https://doi.org/10.1111/j.1469-8986.1993.tb03352.x.
Rhue, Lauren. 2018. “Racial Influence on Automated Perceptions of Emotions.” SSRN Electronic Journal. https://doi.org/10.2139/ssrn.3281765.
Russell, James A. 1995. “Facial Expressions of Emotion: What Lies Beyond Minimal Universality?” Psychological Bulletin 118 (3): 379–91. https://doi.org/10.1037/0033-2909.118.3.379.
Sedenberg, Elaine, and John Chuang. 2017. “Smile for the Camera: Privacy and Policy Implications of Emotion Ai.” https://arxiv.org/abs/1709.00396.
1. EU-funded Horizon 2020 Project SEWA uses FER to improve automated understanding of human interactive behaviour in naturalistic contexts; iBorderCtrl have devised a system for automated border security, which includes FER technology; PReDicT project utilises FER in the medical domain, to improve the outcome of antidepressant treatments.
This publication is a brief report produced by the Technology and Privacy Unit of the European Data Protection Supervisor (EDPS). It aims to provide a factual description of an emerging technology and discuss its possible impacts on privacy and the protection of personal data. The contents of this publication do not imply a policy position of the EDPS.
Issue Author: | Konstantina VEMOU, Anna HORVATH |
Editor: | Thomas ZERDICK |
Contact: | techdispatch@edps.europa.eu |
To subscribe or unsubscribe to TechDispatch publications, please send a mail to techdispatch@edps.europa.eu. The data protection notice is online on the EDPS website.
© European Union, 2021. Except otherwise noted, the reuse of this document is authorised under a Creative Commons Attribution 4.0 International License (CC BY 4.0). This means that reuse is allowed provided appropriate credit is given and any changes made are indicated. For any use or reproduction of photos or other material that is not owned by the European Union, permission must be sought directly from the copyright holders.
ISSN 2599-932X | |
HTML: | ISBN 978-92-9242-473-2 |
QT-AD-21-001-EN-Q | |
doi: 10.2804/519064 |
|
PDF: | ISBN 978-92-9242-472-5 |
QT-AD-21-001-EN-N | |
doi: 10.2804/014217 |